Crop mapping close to real-time in Israel: Development of an artificial intelligence system based on satellite imaging for crop detection
Research team: Dr. Offer Roznation (PI) and Dr. Tarin Paz-Kagan (Co-PI)
​
This research aim is to develop a method for deriving crop maps from a time series of Sentinel-2 images between 2017-2024 to address global challenges in agriculture and food security and assist with police and management of the agricultural area Ministry of Agriculture and Rural Development. This study is the first step towards improving crop mapping based on phenological and textural features retrieved from an object-based time series on a national scale. All major crops in Isreal will be mapped and classified with more than 30 different crops. Different characteristics and inputs of the mean shift segmentation algorithm will be tested to optimize the object-based classification process, including vegetation indices, three-band combinations, and high/low emphasis on spatial and spectral characteristics. Multiply known vegetation indices (VIs)-based time series were tested, together with texture parameters. Additionally, we will compare several widely used machine learning methods for crop classification, support vector machine (SVM), and random forest (RF), in addition to a newer classifier, extreme gradient boosting (XGBoost), and CNN. Lastly, we examined two accuracy measures—overall accuracy (OA) and area under the curve (AUC)—to optimally estimate the accuracy in the case of imbalanced class representation.
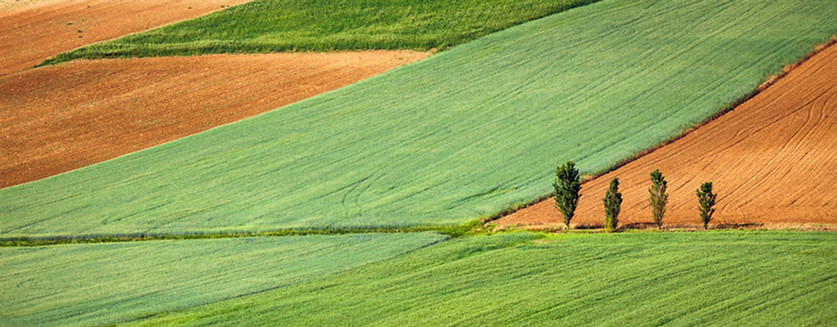

