Using an artificial intelligence-based model to identify the nitrogen state in a plant by spectroscopy to examine the relationships between minerals and metabolites and develop a tool for optimal fertilization in orchards
Research team: Dr. Or Sperling (PI), Prof. Uri Yermiyahu (CI), and Dr. Tarin Paz-Kagan (Co-PI)
MSc:
Nitrogen is a crucial macronutrient for plant growth, development, and productivity. This study aims to develop a real-time approach for diagnosing nitrogen deficiency in orchards using advanced artificial intelligence and chemometrics techniques. Early detection is key to preventing negative impacts on productivity. Implementing an accurate precision nitrogen management model is vital for optimal fertigation, ensuring the right timing, quantity, and application during various growth stages. Real-time nitrogen management enhances resource efficiency and boosts productivity. It achieves this by improving root development, nutrient and water absorption, and increasing resilience to both biotic and abiotic stressors. Our approach includes utilizing unmanned aerial vehicle (UAV) hyperspectral imagery, analyzing non-structural carbohydrate composition through reflectance spectroscopy with partial least squares regression and discriminant analysis, and employing artificial neural network models. The goal is to establish a system that improves resource use efficiency and minimizes environmental impacts. By reducing soil emissions of nitrous oxide and preventing nitrate leaching, we aim to lessen the effects of global warming and groundwater contamination.

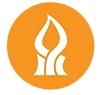
